This may not be a popular opinion (cue comments), but I am not a fan of Machine Learning being used in the security industry.
I'm always skeptical when it seems like the approach is "We don't know how to solve this problem. I know! Let's throw ML at it!!". There are of course niches within security where ML seems to be doing ok-ish, for example detecting malware and financial fraud, but even there, it's used with caution.
Remember that ML is part of the field of statistics: the science of detecting average-case behaviour. In ML you worry about telling the average dog apart from the average cat, and don't worry about the 5% that it gets wrong. Meanwhile security is the field of detecting worst-case adversarial behaviour. In security your system needs to continue being strong even if the attacker can reverse-engineer it and provide worst-case input.
Now consider the paper: "EXPLAINING AND HARNESSING ADVERSARIAL EXAMPLES" by Goodfellow, Shlens, and Szegedy:
Several machine learning models, including neural networks, consistently misclassify
adversarial examples—inputs formed by applying small but intentionally
worst-case perturbations to examples from the dataset, such that the perturbed input
results in the model outputting an incorrect answer with high confidence.
Here is the core graphic from that paper:
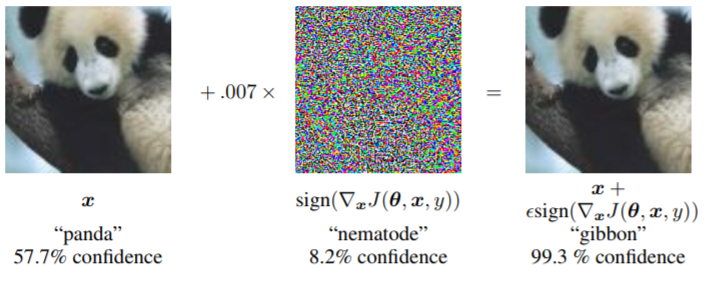
If it's that easy to "hack" an image classifier (arguably the best-researched subfield of ML), then what makes you think you can build an ML-based WAF filter that performs any better against adversarial hackers?
TL;DR: This has been my rant that ML shouldn't have a place in security unless it's by people who really really know what they are doing.